The Most Innovative Things Happening With Background Screening
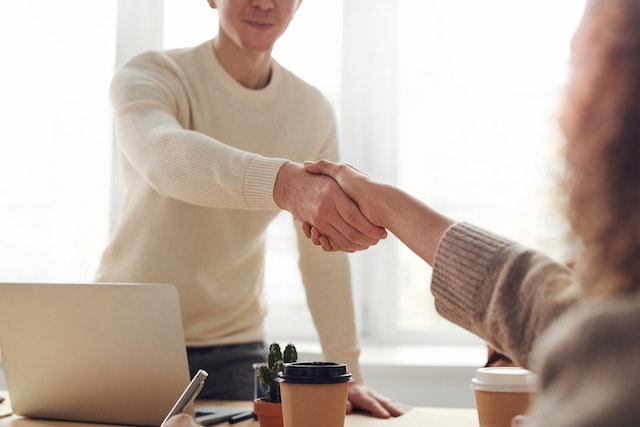
The background screening industry has seen several technological advances recently, including biometric identity verification and robotic process automation. These innovations help background screeners ensure the validity of employment verification data and reduce fraud. Biometric ID verification also helps background screeners provide a frictionless experience for applicants, reducing the likelihood of false applications. Another technological breakthrough is robotic process automation, which automatically allows background screeners to pull employment verification data.
Ai-Powered Background Screening Technology
AI-powered background screening is a growing trend in the workplace. For a background screening company, it is an efficient way to scan substantial data sets to find potential criminals. It is helpful to companies because it is faster than human operators and can analyze vast data sets in less than a second. However, it comes with several risks. It can also put employers and job seekers under pressure. Luckily, there are ways to protect yourself from AI-powered background screening. AI-powered background screening technology can help employers make more accurate hiring decisions. It can quickly scan candidate resumes and pull data on criminal backgrounds, education references, and more. It can also search a decade’s worth of address history and scan documentation to find relevant experience or qualities required for a particular role.
Automated Background Checks
Automated background checks can save companies a lot of time. With these tools, the information about prospective employees can be retrieved in less than a day. This helps HR departments focus on more critical tasks. In addition, companies can quickly run background checks on hundreds of documents. This technology uses secure online platforms and special software to process information.
Many employers have been rethinking the way they handle pre-employment screening. Some companies eliminate the process, while others simply reduce it to meet state and local regulations. However, this can leave employers vulnerable to claims of negligent hiring.
Automated background checks can save companies a lot of time and money. Manual background checks require enormous labor, which increases with the volume of orders. However, it can be risky and expensive to scale labor. Eventually, this can lead to inefficiency and lower profits.
Ai Filters That Avoid Bias In The Hiring Process
AI filters can be used in the hiring process to help managers make better decisions about candidates. For example, consider a company looking to hire a new management role. Two candidates are vying for the position. One is female, and one is a minority. The hiring manager will make the final decision. In the hiring process, he will assume that the female candidate prefers a part-time schedule.
A major company realizes its past hiring practices have been biased against women and would benefit from having more female candidates in the management pipeline. Using AI, the company can analyze job postings to spot gender-biased language. As a result, it can post jobs in gender-neutral languages, which increases the number of female applicants. AI can also help companies write inclusive job descriptions. This will make the hiring process more equitable and reduce implicit bias.
Additionally, AI talent matching tools can reduce guesswork and preference. In addition, applicant tracking systems can help prevent bias in the hiring process. These systems allow companies to review multiple applicants at once, which is a prime time for implicit bias.
Ai Filters That Provide Real-Time Alerts On Newly Completed Screenings
Real-time alerts from AI-based background screening platforms help employers monitor potential hires for various risks. With the help of machine learning algorithms, these tools can identify the context of any activity and provide alerts based on their significance. These alerts can be low-level, like a patient forgetting routine activities, or high-level, like a potential fall.
Other alerts rely on more data and statistics. These are similar to the alerts produced by the faster-running models. Finally, those with intermediate alerts are similar to volume-confirmed alerts but may take longer to report valid changes in trends. These alerts are analogous to monitoring the moving average.